Why trying to "shape" AI innovation to protect workers is a bad idea
Instead, we should empower workers and create mechanisms for redistribution.
I’ve been to a number of meetings and panels recently where intellectuals from academia, industry, media, and think tanks gather to discuss technology policy and the economics of AI. Chatham House Rules prevent me from saying who said what (and even without those rules, I don’t like to name names), but one perspective I’ve encountered increasingly often is the idea that we should try to “shape” or “steer” the direction of AI innovation in order to make sure it augments workers instead of replacing them. And the economist Daron Acemoglu has been going around advocating very similar things recently:
According to Acemoglu and [his coauthor] Johnson, the absence of new tasks created by technologies designed solely to automate human work will…simply dislocate the human workforce and redirect value from labour to capital. On the other hand, technologies that not only enhance efficiency but also generate new tasks for human workers have a dual advantage of increasing marginal productivity and yielding more positive effects on society as a whole…
[One of Acemoglu and Johnson’s main suggestions is to r]edirect technological change to enhance human capabilities: New technologies, particularly AI, should not focus solely on automating tasks previously done by humans and instead seek to empower and enable workforces to be more productive.
So I thought I’d write a post explaining why I think this is generally a bad idea — and what we should do instead.
In theory, new technologies can have several different effects on human workers. They can:
reduce demand for human workers by replacing their labor,
increase wages by making human workers more productive at their jobs,
create new demand for new kinds of jobs, and
increase overall labor demand through economic growth.
In addition, new technology can affect inequality by favoring low-skilled workers or high-skilled workers.
It’s understandable that we might want to steer or shape the development of AI technology so that it maximizes the benefits for workers, and avoids the “replacement” part. But there is a big problem with the idea — namely that no one, including Daron Acemoglu or any economist, has any idea how to predict which technologies will augment humans and which will simply replace their labor.
Imagine yourself in 1870 — on the eve of what we call the Second Industrial Revolution. And suppose that you had foreknowledge of all the marvelous technologies that would be either introduced or greatly expanded over the next half century — mass production, railroads, electricity, automobiles, telegraphs, telephones, water supply, airplanes, and so on. Suppose someone gave you the chance to decide which of those technologies you thought would displace human workers, kill jobs, and decrease wages, and which you thought would augment human workers, create jobs, and raise wages? Which would you put in each category?
In fact, this would have been an incredibly hard prediction to make, even if you had all of the data and tools available to a modern economist. For any one of those technologies, you could name specific people whose jobs might be disrupted. Cars would disrupt the jobs of everyone involved in taking care of horses. Mass production would disrupt the jobs of artisan manufacturers. Electric lighting would disrupt the jobs of people who produced whale oil for lighting, and so on.
But that would tell you little, because there would also be a bunch of jobs created by each of these technologies — car factory workers, machinists, power station workers, and so on. In 1870 you could sort of imagine what those new jobs might be, but you wouldn’t know for sure, nor could you know how many of them there would be or how they might pay. You could write out a mathematical model like Acemoglu’s, and yet without knowing the parameters of that model, you wouldn’t be able to use the model to predict whether any particular industrial technology would displace or augment human labor on net. And because every technology is different, you couldn’t calibrate the parameters for the model on historical data for old technologies.
You would have no choice but to rely on judgement and gut instinct.
Anyway, looking back with the benefit of a century and a half of hindsight, we know that the technologies of the Second Industrial Revolution, overall, turned out well for workers. In 1970, pretty much every human being was still working, and at much higher wages and living standards than in 1870.
Labor’s share of income fluctuated a bit, but stayed pretty high:
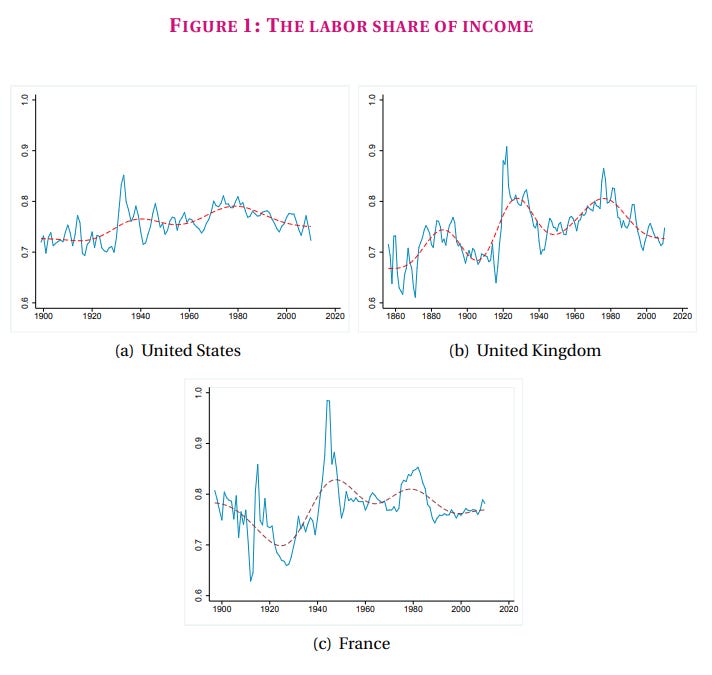
Income inequality also fluctuated somewhat, but overall it stayed the same in North America and fell in Europe:
Overall, looking at this historical record, you might reasonably conclude that trying to intentionally slow down the progress of industrial technology would have been a bad idea.
But even knowing that this was the (happy) outcome of the Second Industrial Revolution in the aggregate, it would be hard to know, looking back from 1970, whether any specific industrial technologies ended up being bad for workers. We can certainly list lots of jobs that were created and destroyed — by 1970 we had a lot more office workers and a lot fewer farm workers, for instance. But isolating the impact of each specific technology on the whole zoo of jobs and wage levels in the economy would be a daunting task for any empirical economist, even with a full historical dataset.
And if it would be nigh impossible in hindsight, it would be utterly hopeless looking forward in 1870. Yet this impossible task is exactly what would be required of any economist, historian, science fiction author, government worker, or economics blogger standing in 2023 and trying to predict the impact of specific AI technologies on jobs and wages over the next 50 or 100 years.
Models don’t help — as I mentioned, their predictions would be totally dependent on parameter values that can’t be estimated from past data. Experiments won’t tell us much either — so far, studies by Brynjolffson et al. (2023), Noy and Zhang (2023), and Peng et al. (2023) have found that generative AI improved human workers’ capabilities in a variety of fields, but this doesn’t tell us much about whether that would lead to employers hiring more of those workers or fewer, or what new jobs the AI tools might enable, or what they’d do to economy-wide demand.
So if we were to set up a panel of experts and task them with deciding which lines of research and innovation to encourage and which to discourage in order to maximize jobs and wages, they would be operating purely on gut instinct and quasi-science-fictional supposition.
So far, the guesses of experts have proven to be little more than shots in the dark. Seven years ago, Geoffrey Hinton, one of the pioneers of AI, said:
“I think if you work as a radiologist, you are like the coyote that’s already over the edge of the cliff but hasn’t yet looked down…People should stop training radiologists now. It’s just completely obvious within five years deep learning is going to do better than radiologists…It might be 10 years, but we’ve got plenty of radiologists already.”
Six years later, how did Hinton’s confident prediction turn out? In 2022 there was a global radiologist shortage, and radiology businesses were begging the U.S. Congress for more money to train radiologists. As of 2023, medical imaging vacancy rates were at all-time highs.
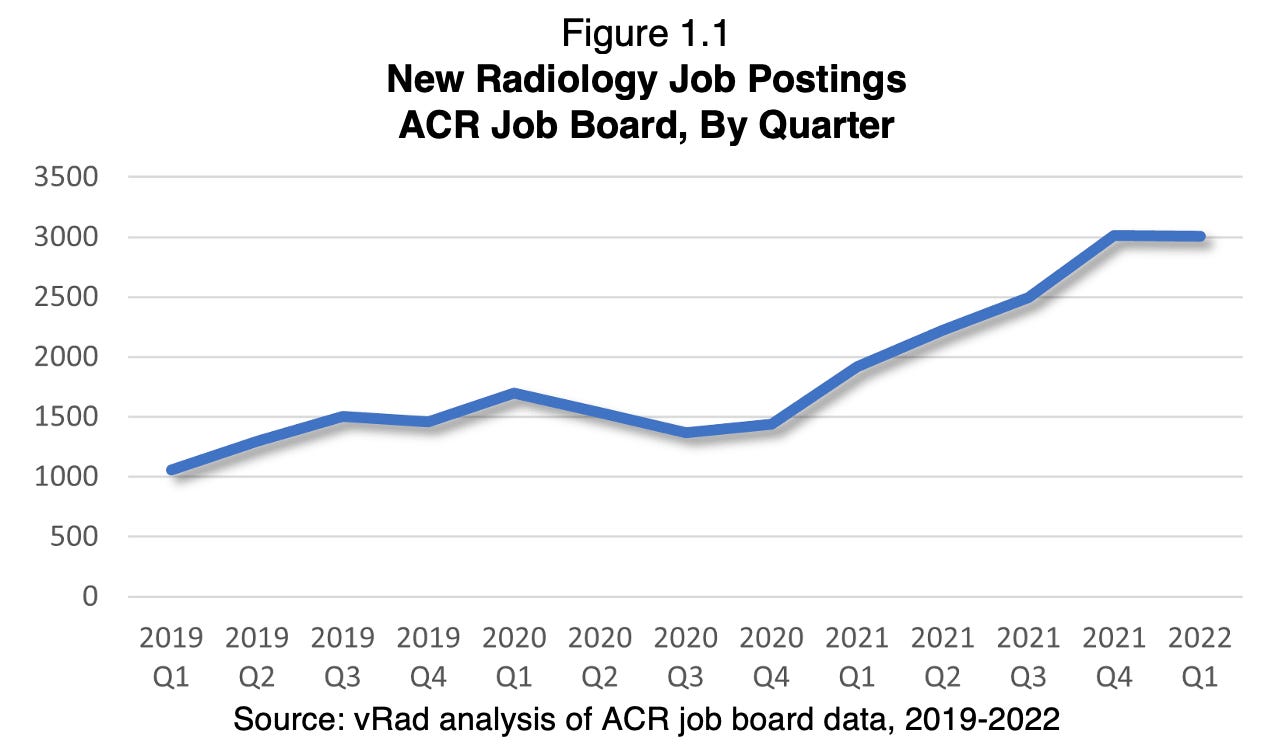
And radiologist salaries were high and rising:
It's no surprise that with strong demand for radiologists, compensation increases have followed. That includes high offers for new radiologists (radiologists earn the fifth highest starting salaries of all specialties) and signing bonuses of $10,000 to $50,000 according to the Merritt Hawkins report. Radiologists are also receiving “stay bonuses” and pay increases from their current employers in an effort to increase retention.
Someday, Hinton’s prophecy of the replacement of radiologists might come true. Or maybe it never will. But within the time frame he specified, his guesses were absurdly off the mark.
And that’s pretty much how it’s going to go with any expert panel or regulatory commission that the government sets up to restrict or accelerate various AI applications or capabilities. Yes, the panels will be diverse, with historians and sociologists and economists as well as computer scientists and engineers. But there is no reason to believe that their collective gut instinct will be any more accurate than Hinton’s. The question of the future economic impacts of yet-to-be-discovered technologies is simply a question on which no human expertise is likely to exist, either individually or in aggregate.
So in practice, any panel or commission set up to speed up and slow down various types of AI will be simply adding noise to the innovation process, offering rewards and punishments essentially at random. That’s not good for the development of technology as a whole, since it introduces uncertainty into the innovation equation. But it’ll also be ineffectual in terms of actually protecting human workers.
So what should we do? One option is to just do nothing and to let innovation take its course, and then try to figure out later what the problems were and try to fix them. I expect some countries will follow this approach, and in general I think this isn’t a bad idea. If we see jobs being destroyed en masse, we can always start taxing AI or raising corporate taxes or subsidizing human labor or implementing universal basic income or whatever, before things get really dire. This is pretty much what we did with the Second Industrial Revolution, when we implemented new taxes, welfare states, and labor regulations in response to high inequality in the early 20th century.
But in fact I think we can probably do better than that. Instead of trying to slow down technologies that sound scary, or waiting to clean up problems after they happen, we can improve the robustness of our institutions now. With more robust institutions, we can minimize any negative technological impacts on human workers, and reduce the amount of “cleaning up” we need to do later.
One important institution is labor power. Right now, for example, dockworkers’ unions in the U.S. are opposed to the automation of ports. The same was true in the Netherlands, where Rotterdam has been trying to build the world’s most automated port. But eventually union fears were quieted, and arrangements were made to ensure that dockworkers would still have work. A more harmonious relationship between business, government, and labor enabled unions to — reluctantly, warily — take the long view. Meanwhile, in Germany, the story is similar — in a land where unions have seats in boardrooms, they also tend to support automation technology, because they understand that these technologies help the whole enterprise grow and stay competitive.
Some technologists will simply assume that unions will be eternal enemies of automation, and that technology can only progress over labor power’s dead body. But I think the experience of Northern European countries shows that when unions are given a long-term stake in the growth and competitiveness of their companies, their interests become much more aligned in favor of embracing new technology.
A second important institution is the welfare state. If AI does destroy jobs or wages, it’s going to be so that someone — business owners, but also some kinds of high-end workers — will make more money. A welfare state can’t prevent every negative outcome for every individual, of course, but it can strongly mitigate any overall disruptive impact of technologies, through taxes and government benefits. The introduction of simple, near-universal cash benefits would be an important way of insuring against any sudden disruptive job market impacts from AI; if things suddenly started getting bad, it would be a lot easier to scale up this sort of program than to make it from scratch on the fly. Also, corporate taxes could be modified so if the labor share of income gets too low, the tax rate on profits automatically goes up, with the proceeds going to cash benefits.
Anyway, at the events I recently attended, I did hear people tossing out some ideas like these which is good. I would urge everyone in and around the AI policy space to steer their thinking away from the idea that they can predict the economic impacts of future technologies, and toward the development of economic institutions that will minimize any downsides. Let’s stick with what worked in the last industrial revolution, and not try to be sci-fi prophets.
The article assumes that AI can replaces workers just by introducing some "clever" IT processing, which is what current AI can do, but not much else. In fact, what is called "AI" today is simply a better way of solving certain programming issues that are not possible with traditional programming. Image Processing, for example, provides ability to classify and identify images, but the final decision must be human, as the case with radiologists proves - they use "AI" to analyze images, but judge the outcome and re-check based on their experience. The result: they can serve more people, they can allocate more time per case, and they can allocate time to identify new phenomena not part of the trained model, which is outside the abilities of the AI solution. This will be the case in most areas: Workers will be able to allocate more time to thinking, attention, care, and less on robotic tasks (think of customer support, being able to listen instead of search through mountains of documentation). The current wave of AI, mostly bringing NLP and machine vision to the forefront is just correcting a decade long deterioration in the quality of traditional solutions, bad app, unreliable backends etc. We need it, we are tired of low quality software. Nothing to be afraid of.
Yes! Yes! Yes! We should want and encourage automation to automate people out of jobs, particularly jobs that suck. AND we need to create institutions that ensure people still thrive and can pursue activities that can lead to a better world without worrying if they can have food on their tables.