At least five interesting things to start your week (#2)
Useless student loans, the U.S. factory-building boom, middle-aged Millennials, opportunity for the poor, and limits to AI
This is the second of my weekly roundups! I’ve decided to start numbering them, like Packy McCormick does with his “Weekly Dose of Optimism” posts, so they don’t all have the same title. I’m also going to start putting links that I bump up from the comments in the next week’s post, so that people who read Noahpinion via email (i.e. most of you) will see them. For now, I’m going to keep 2 of the 5 items free, and paywall the others.
If you have any thoughts on these ideas, or other suggestions for how I could improve these roundups, please let me know!
Anyway, on to this week’s Five Interesting Things. As usual, if you have any more you’d like me to check out, please leave them in the comments.
1. New research suggests that government-provided student loans aren’t helping students
Student lending used to be partly public and partly private, but since the 2008 financial crisis it has been almost entirely taken over by the government. This is why student debt has emerged as such a policy flashpoint in recent years, with calls for student debt forgiveness, pauses on loan repayments, and changes in repayment going forward. But one additional issue is how much the U.S. government should lend to students in the first place.
The main argument in favor of government lending to students is about borrowing constraints. If disadvantaged students A) can’t pay for school without a loan, and B) can’t get private banks to lend them money, then government loans will mean a lot more disadvantaged students who get to go to college and/or graduate school. Which presumably will mean less inequality and more opportunity, which are things we all should want.
But a new paper by Sandra Black, Lesley Turner, and Jeffrey Denning suggests that it doesn’t tend to work out this way in practice — at least, not as far as graduate school is concerned. Using data from Texas, the authors study a policy change in 2006, where the federal government effectively uncapped the amount that grad students were able to borrow from it. This was called the Grad PLUS loan program. They found that students did borrow more money after the change. But this didn’t lead to increased enrollment in grad programs, either for underrepresented minorities or overall:
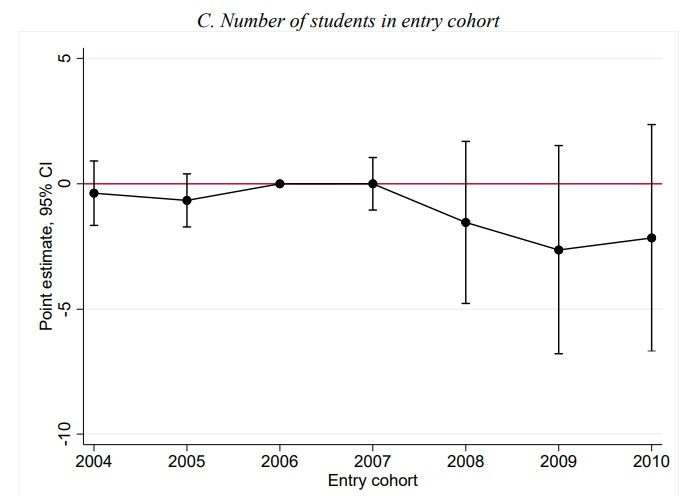
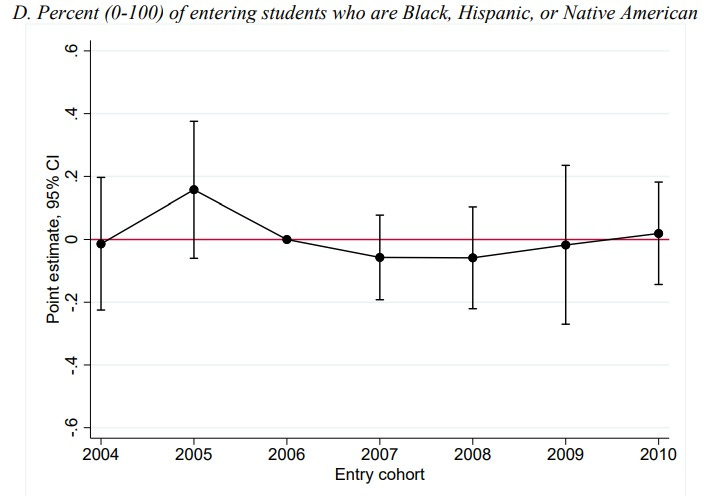
Nor did it increase degree completion rates, even ten years later, even for students who were financially constrained and who thus should have been helped by the loans:
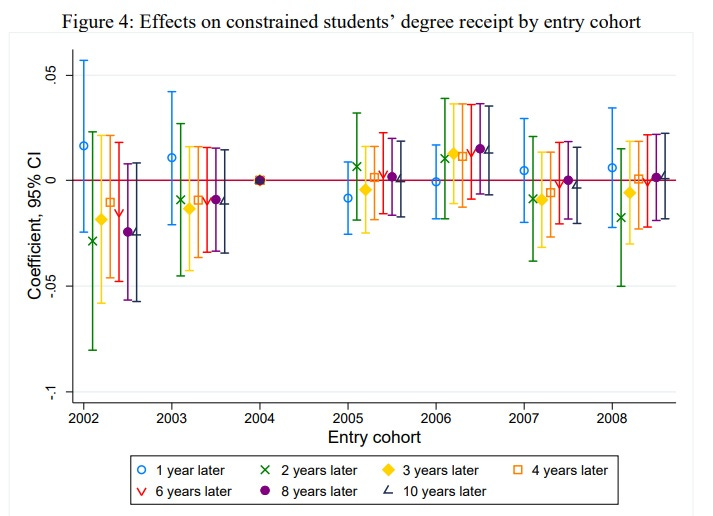
But the increased borrowing did have one big effect (other than making students more indebted). It increased tuition. In fact, it increased tuition by pretty much exactly the same amount that borrowing increased:
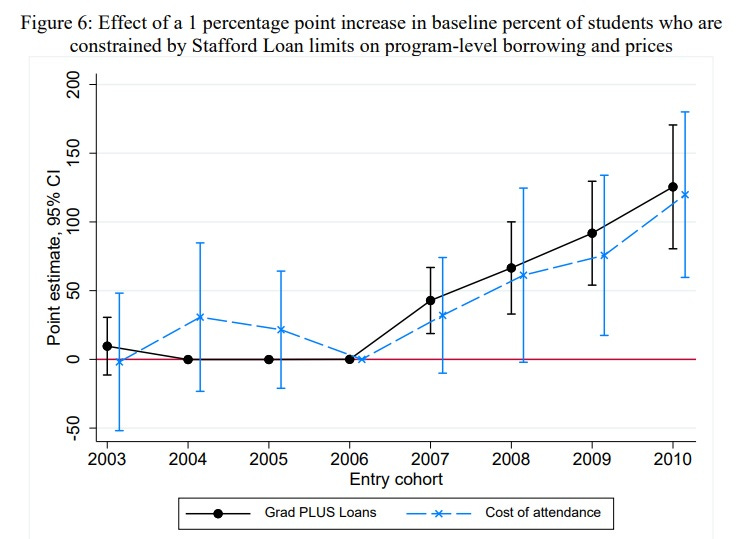
This should be a huge wake-up call for people who think the U.S. government should lend more to students. It supports the theory that all government loans do is to drive students deeper into debt and push up the price of education, without helping more disadvantaged Americans get degrees. If this research is correct, these loans are basically just draining money from disadvantaged students’ futures, and putting it directly into the pockets of educational institutions. That’s not something our government ought to be doing.
2. America is experiencing a factory-building boom
How can we know if industrial policy is working? Well, one way would be if we actually start making more of the stuff that industrial policy tries to produce — more solar panels, semiconductors, electric cars, and so on. But it takes time to ramp up production, so in the meantime, we can look at how many factories are being built to make these things. And here, the U.S. seems to be getting pretty immediate and impressive results! Inflation-adjusted construction spending in the manufacturing industry has absolutely skyrocketed since June 2022, from $90 billion to $189 billion:
That’s an incredible amount. Factory construction spending more than doubled in one year, after being essentially constant for decades. And it perfectly lines up with the passage of the CHIPS Act in July 2022 and the Inflation Reduction Act in August 2022. In fact, big investments by companies like Intel and Ford — making chips and EVs — figure prominently in these numbers.
And even more incredibly, this is happening at a time when construction spending in pretty much every other area of the economy is falling in inflation-adjusted terms!
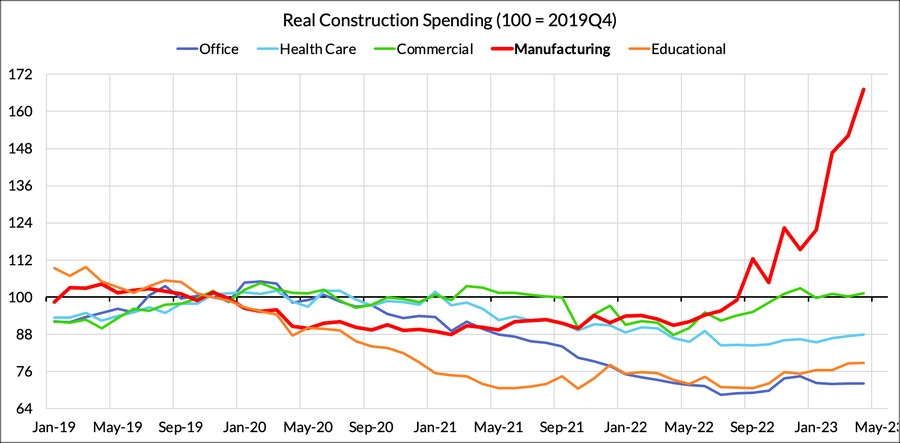
And the spending is spread very evenly around the country, with big increases in every region except the Northeast.
Anyway, this is pretty strong preliminary evidence that industrial policy works. American companies are perfectly willing to spend big sums on factories if they get a little push. The danger, though, is that this spending will ultimately translate into only a modest amount of actual new manufacturing capacity — the money could get eaten up by delays related to NEPA and other legal holdups, and frittered away on government-mandated side goals. Time will tell. But spending money on factories is a very important and necessary first step toward the dream of making America a manufacturing superpower once again.
3. Millennials are getting a lot more comfortable and a bit more conservative
Back in April I wrote a post called “Will there be a Millennial Big Chill?”. I observed that as the Millennial generation eases into middle age, their income is rising strongly and has surpassed that of previous generations at a similar age, and their wealth and homeownership are closely tracking that of previous generations. And therefore I wondered if my generation would eventually become, if not more conservative, at least calmer and more satisfied with the status quo. Well, a recent post by Jacob Zinkula adds some more bits of data to support the hypothesis that Millennials are financially healthy:
[T]he median salary of an individual US adult aged 25 to 34 is $52,156. The median salary of an adult aged 35 to 44 is $62,444. At this point, millennials will be anywhere from 26 to 42 years old…
The average US millennial's net worth more than doubled between the first quarter of 2020 to $127,793 as of the first quarter of 2022…
By the end of [2022], a majority of [Millennials] — about 51.5% — owned a home…The average millennial was 34 years old when the generation reached this milestone. Gen X and Boomers were 32 and 33 years old respectively when their generations became majority owners.
Compare those salary numbers to the median personal income of $37,522, and Millennials aren’t doing half bad!
Zinkula goes on to note one major disadvantage of Millennials relative to earlier generations — they have more student debt at a similar age. Of course, student debt is included in the net worth numbers, and Millennials have the same net worth as their parents did at their age. But for the same amount of wealth, it’s worse to have more debt, since it constrains you to make interest payments every month, which makes it harder to switch jobs or start a business. Thus, student debt is the one remaining scar that Millennials bear from the Great Recession. But all in all, they’re doing financially fine.
As for whether this newfound wealth will make Millennials turn more conservative, Nate Cohn has a recent analysis in the NYT suggesting that the oldest cohort of the generation already starting to vote a bit more Republican:
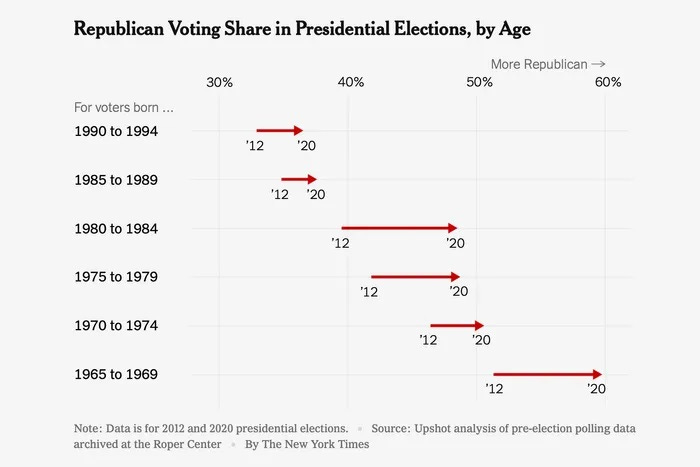
Interestingly, the oldest Millennials are the ones who got the most screwed by the Great Recession; if you were born in 1984, you were 24 when the crash hit. So it’s interesting that they’re also showing the biggest conservative shift. It could be an age thing — Millennials might simply be slightly later in following the well-trodden path of becoming more conservative as they become homeowners and parents. Or it could be a generational thing, with older Millennials being more culturally similar to Gen Xers, and less defined by social movements like BLM. Obviously progressives hope for the latter; only time will tell.
In any case, I don’t expect Millennials to become a bunch of Republicans, at least not soon. But I do think these are a few more small signs that a generational Big Chill is on the way.
4. A social safety net improves equality of opportunity
We Americans traditionally like to think that we embrace equality of opportunity. Unlike the class-based societies of Old Europe, everyone in America is supposed to have the chance to pull themselves up by their bootstraps, no matter how humble their background. So it’s kind of depressing that fewer people tend to rise out of poverty in the U.S. than in other rich countries. This is from a new working paper by Parolin et al:
This study investigates differences in intergenerational poverty in the United States (U.S.), Australia, Denmark, Germany, and United Kingdom (UK) using administrative- and survey-based panel datasets…Intergenerational poverty in the U.S. is four times stronger than in Denmark and Germany, and twice as strong as in Australia and the UK.
That’s bad news! The next question is why the U.S. is so far behind here. The authors spend most of the paper checking out various factors that might be causing the differences in poverty persistence, using various data sets. Some factors they test are:
Family background — i.e., a two-parent home, mother’s educational attainment level, etc.
Racial discrimination
Geographic disadvantage
“Mediating benchmarks” — things like whether someone gets a degree, gets a job, gets married, etc.
Taxes and government transfers
Interestingly, they find that the only factor that explains a major chunk of the U.S. disadvantage is the last one. Poor American kids are much more likely to grow up to be poor than kids in other rich countries, regardless of their race, place of birth, family background, or whether they follow a “success sequence” in their early life. That’s not to say things like family background and getting an education don’t matter — they do. The U.S. just doesn’t look worse in these dimensions than the other countries.
The only big explainable difference that the authors find between the rich countries is taxes and transfers. In places like the UK and Australia, taxes and the welfare state help give some poor people a boost out of poverty. In the U.S., these mechanisms are less present. The authors reckon that this explains about a third of the international difference in poverty persistence.
Of course, that leaves the remaining two-thirds unexplained. The authors politely call this a “residual”, but what it really means is that they have no idea what’s causing the majority of the U.S.’ greater poverty persistence. As with so many things in economics, this one remains a mystery for now.
But while most of the U.S.’ opportunity problem remainsunsolved, this paper does tell us one important thing: A strong welfare state helps. Conservatives have told us for many years that welfare traps poor people in a cycle of poverty; this paper is one more piece of evidence that the opposite is true.
5. Limits to generative AI?
Amid all the frenzied discussion of how to limit AI risk, how to prevent AI from taking people’s jobs, and how to use AI to make money, there has been relatively little attention paid to what I think is a fairly important question: What if generative AI progress slows down fairly soon? After all, technologies tend to be S-curves rather than true exponentials — at some point, rapid breakthroughs tend to taper off.
I’ve recently read a few arguments making the case that the extraordinary explosion of capabilities represented by the release of OpenAI’s ChatGPT and GPT-4 over the past year is simply the steepest part of an S-curve, and that soon we’ll see a slowdown to more incremental levels of progress. One is this blog post by Dylan Patel, arguing that AI’s computational costs are going to put a hard limit on AI progress by making both the electricity and the chip requirements prohibitively expensive. He writes:
Costs for training for these civilization-redefining models have been ballooning at an incredible pace. Modern AI has been built on scaling parameter counts, tokens, and general complexity an order of magnitude every single year…
Regarding parameter counts growth, the industry is already reaching the limits for current hardware with dense models—a 1 trillion parameter model costs ~$300 million to train…Another order of magnitude scaling would take us to 10 trillion parameters. The training costs using hourly rates would scale to ~$30 billion…[T]raining this model would take over two years. Accelerator systems and networking alone would exceed the power generated by a nuclear reactor. If the goal were to train this model in ~3 months, the total server Capex required for this system would be hundreds of billions of dollars with current hardware.
This is not practical[.]
Of course, one way to reduce these costs is to make better computer chips and more efficient methods of using those chips to train the models. In another recent post, Brian Chau argues that improvements of this type are slowing down — not quite a slowdown of Moore’s Law, but something sort of analogous, with the incremental gains from parallelizing computation on GPUs and other AI-specialized chips becoming slower and more expensive over time.
A second possible reason for slowing progress would be that LLMs could run out of good training data. Remember that these models basically read everything that has ever been written, and try to extract patterns from all that text that give it an idea of how to construct human-sounding speech. But once you’ve read all there is to read, you eventually hit a wall in terms of how much additional benefit you can get from simply encoding that information in an even bigger set of parameters.
This is old news at this point, but a paper by Villalobos et al. from October 2022 warns that high-quality English-language data will be exhausted in just a couple of years:
We investigate the growth in data usage by estimating the total stock of unlabeled data available on the internet over the coming decades. Our analysis indicates that the stock of high-quality language data will be exhausted soon; likely before 2026. By contrast, the stock of lowquality language data and image data will be exhausted only much later; between 2030 and 2050 (for low-quality language) and between 2030 and 2060 (for images). Our work suggests that the current trend of ever-growing ML models that rely on enormous datasets might slow down if data efficiency is not drastically improved or new sources of data become available.
There’s still plenty of “low-quality data” — Harry Potter fanfic and the like — but it may not make LLMs able to speak any better. If low-quality data doesn’t add much, we could be looking at an LLM slowdown pretty soon here.
If data and compute — the fundamental resources of AI — are limited, researchers will have to resort to other methods to improve performance. One is reinforcement learning from human feedback, also known as RLHF. But it’s not yet clear if humans actually make AI much better by giving it feedback; they might even make it worse.
Of course that leaves all sorts of other ideas to try — inventing newer hardware and ways of making hardware run faster, incorporating different kinds of data into LLMs, looking for newer and better algorithms, or even just investing more money. Despite the dramatic language in some of these posts, I don’t think that anyone really thinks generative AI is about to suddenly hit a brick wall. But the amazing leap of progress that happened in late 2022 might end up being an unusual event rather than a new normal. Already, OpenAI says they’re not thinking of training a GPT-5 “for some time”.
Obviously I am not qualified to adjudicate this debate, or predict the rate of AI progress. But I think both technologists (including investors) and policymakers need to at least consider the possibility that a slowdown will happen. That possibility should make us cautious about clamping down on AI progress with harsh regulation. And we also can’t put all our hopes — or all our resources — in this single vector of technological progress. We should simultaneously be investing in lots of other vectors — biotech, energy, space, and all the rest. The idea that exponentially exploding AI will soon make everything else irrelevant remains, for now at least, in the realm of science fiction.
"Of course that leaves all sorts of other ideas to try — inventing newer hardware and ways of making hardware run faster, incorporating different kinds of data into LLMs, looking for newer and better algorithms, or even just investing more money."
The gains we are seeing with the open source LLMs, LlaMa, Vicuna, Wizard, et. al. with the 7B, 13B and 65B parameter models that can be run at home is where the action is. Spinning up your or your companies own custom LLM or large multimodal model (LMM) on run-of-the-mill GPUs or some AWS compute for a day or two is equivalent to the explosion of the WWW in 1996. As for running out of training data, Whisper by OpenAI, which offers an order of magnitude more training data by introducing voice, TV shows, radio broadcasts, YouTube videos, etc. into the mix will push back the "running out of training data based on text" by at least a year or two. Add in sparse neural nets and other technologies that introduce orders of magnitude more efficiency with current data sets, and the widespread adoption of custom hardware, and we have A.I. (M.L.) liftoff in all fields of human knowledge, including M.L. itself.
In his interview with ilya sutskever, Jensen Huang said he was looking forward to using hardware acceleration to make AI compute costs a million times cheaper.